
Baseline qualification framework including feedstock characterization, in-situ process monitoring, defect formation, and effect-of-defects. A predictive capability for properties and performance across AM systems reduces the overall data burden and qualification timeline.
Problem
A major limiting factor for the adoption of AM parts for structural applications is the challenge of qualification. The wide range of AM process types, equipment suppliers, manufacturing parameters, and feedstock formulations makes each system unique and subject to its own qualification protocol. Often, this extends even to the same model of system in a different location. This highlights the need for a qualification process that is based on fundamentally important variables rather than simply those that are easy to identify.
Objective
Building off the efforts of Phase 1, this project is advancing a data-driven qualification approach that allows relationships across platforms and alloy systems to be inferred using intelligent machine-learning algorithms and physics-based modeling. This can allow predictions to be made across AM systems, which can reduce the amount of data needed to qualify a new machine and certify a new part, greatly speeding the adoption of AM parts into military vehicles.
Technical Approach
Working closely with collaborators at the U.S. Army Combat Capabilities Development Command (CCDC) Ground Vehicle Systems Center (GVSC), an interdisciplinary team of faculty and students from the Colorado School of Mines, University of Colorado, and the University of Pittsburgh are using process sensors, design optimization, materials science, physics-based modeling, and mechanical testing combined with machine learning to develop strategies to implement AM faster and more efficiently within the U.S. Army’s ground vehicle fleet. The finite element analysis from Phase 1 is being extended to include thermal loading, as well as more clearly defined mechanical loading. These multi-factor models will be validated via part fabrication and testing. Collection and analysis of test data from samples made on a variety of machines and process parameters will continue and further advance the training of the machine learning algorithm that will be used to relate material properties to machine and processing conditions. A common data schema will be implemented that tracks the relevant process and monitoring information to enable transfer learning across different Laser Powder Bed Fusion (LPBF) systems with the same feedstock. An extensible cyber-physical strategy based on the recommendations from Phase 1 will be piloted.
Project Participants
Project Principal
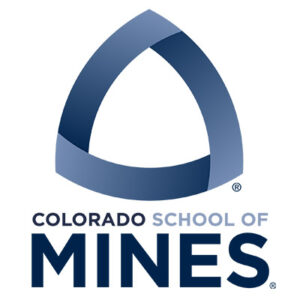
Other Project Participants
- Army Ground Vehicle Service Center
- Colorado State University
- University of Pittsburgh
Public Participants
- U.S. Department of Defense