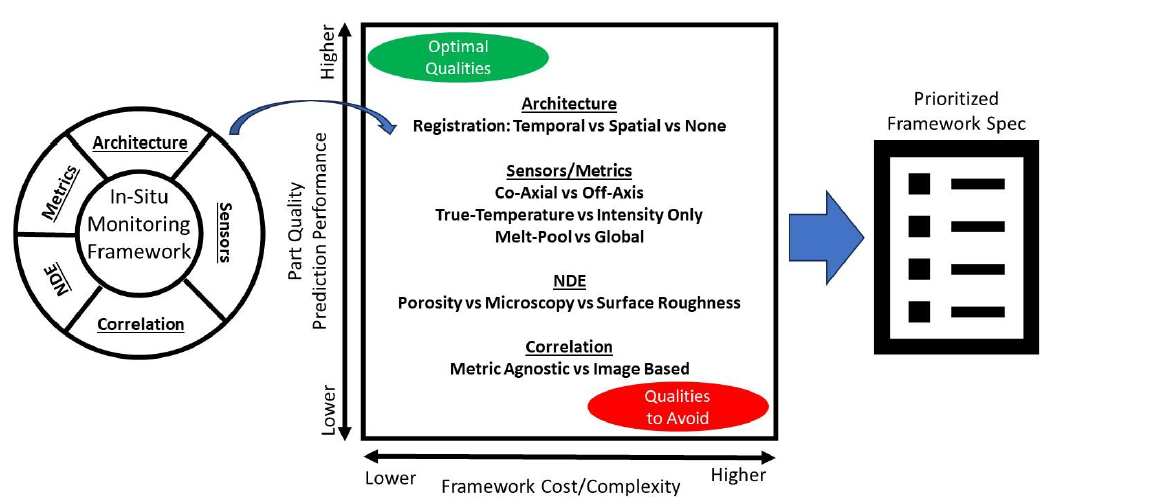
Artificial intelligence (AI) will evaluate datasets to determine an optimal in-situ monitoring framework while balancing cost and complexity with part quality predictive performance resulting in a prioritized in-situ monitoring specification.
Problem
Laser Powder Bed Fusion (LPBF) and Directed Energy Deposition (DED) additive manufacturing (AM) processes have the potential to reduce Department of Defense (DoD) and industrial sustainment costs and delays via on-demand part production and repair. LPBF and DED part quality is dependent on in-process variables requiring significant post-build inspections adding cost and schedule to builds offsetting the benefits of these AM processes. An in-situ process monitoring framework with the ability to predict post-build outcomes with high statistical accuracy is needed.
Objective
The goal of the project is to foster conditional inspection practices by evaluating different in-situ monitoring framework qualities and features for their ability to predict post-build non-destructive examination (NDE) outcomes with high statistical confidence and use the results to develop a framework specification for in-situ monitoring.
Technical Approach
The project will evaluate three different artificial intelligence (AI) correlation applications: Artisan-AI©, Pyramid Ensemble Convolution Neural Network (PECNN), and Ultralytics YOLOv8 on their ability to predict post-build part inspection outcomes on two existing data sets. The project team will develop requirements and metrics for evaluating the AI applications and extract metrics from NDE results and sensor data from the two existing datasets. With metrics established, both datasets will be evaluated by the three AI correlation applications to determine a predicted NDE result. Results will be reviewed to determine which features of the dataset using which AI correlation application produced the highest confidence NDE prediction outcomes. From this analysis, a draft in-situ monitoring specification will be delivered.
Project Participants
Project Principal

Other Project Participants
- NCDMM/America Makes
- The Ohio State University
- University of Louisville
- Macy Consulting, LLC
- Air Force Research Laboratory
Public Participants
- U.S. Department of Defense